Introduction
Artificial Intelligence (AI) has attracted considerable attention from Civil Engineers over the last decade. However, traditionally, AI has been trained using input and output datasets with simple loss functions, which incorporate no physical system knowledge into the learning process, while requiring huge amounts of data, which are either costly to produce or unavailable. Our research will integrate fundamental physics into the training process of deep learning processes, engaging computational modelling and deep neural network, and establish a new generation of physics-informed deep learning methods.
​
Physics-informed deep learning (PIDL) is a recent interdisciplinary research area that combines the principles of deep learning with the fundamental laws of physics to design and develop accurate and efficient machine learning algorithms for scientific and engineering applications. PIDL involves training deep neural networks with partial differential equations (PDEs) as part of the loss function, which enables the network to capture the underlying physical properties of the system being modeled. This approach allows for the integration of prior physical knowledge into the machine learning process, leading to more accurate predictions and improved generalization capabilities.
​
PIDL has been successfully applied in a range of scientific and engineering fields, including fluid mechanics, material science, and image analysis, to name a few. By leveraging the physics of the underlying system, PIDL algorithms can learn from fewer data points and reduce the computational cost associated with traditional machine learning methods. PIDL has the potential to revolutionize the field of scientific computing and enable more efficient and accurate predictions of complex physical phenomena.
​
Related research can be divided into:
Part 2. Physics informed deep learning for groundwater prediction
Part 1. Integrating coupled simulation of surface water and groundwater with artificial intelligence
Mr Jianwei Zhang, PhD Candidate
Water resource system is affected by many uncertain, high complexity, non-stationarity, dynamism and non-linear factors. This research will integrate coupled simulation of surface water and groundwater with artificial intelligence to improve the prediction accuracy of the coupled model and reduce the calculation load.
​
To predict daily runoff, most of the existing studies focused on the model structure and the computational load, without considering the impact from the selection of multiple input variables on the model prediction. This article presents a study to evaluate this influence, and provides a method of identifying the best meteorological input variables for a run off model. Rainfall and multiple meteorological data have been considered as input to the model. Principal Component Analysis (PCA) has been applied to the data as a contrast, to reduce dimensionality and redundancy within this input data. Two different deep RNN models, a long-short term memory (LSTM) model and a gated recurrent unit (GRU) model, have been comparatively applied to predict runoff with these inputs. In this study, the Muskegon river and the Pearl river were taken as examples. The results demonstrate that the selection of input variables have a significant influence on the predictions made using the RNN while the RNN model with multiple meteorological input data is shown to achieve higher accuracy than rainfall data alone. PCA method can improve the accuracy of deep RNN model effectively as it can reflect core information by classifying the original data information into several comprehensive variables.
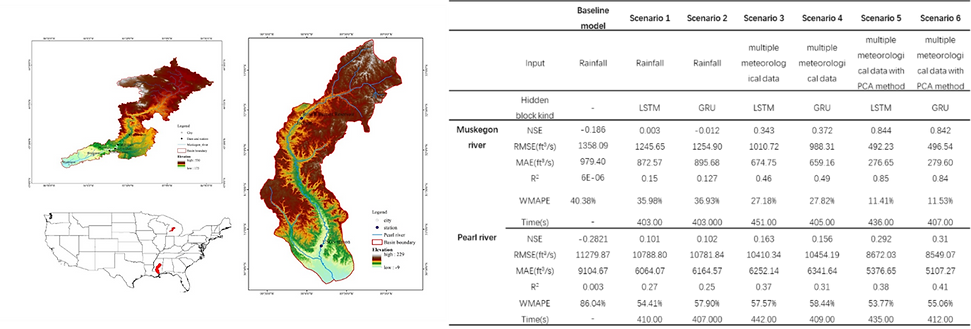
Part 2. Physics informed deep learning for groundwater prediction
Mr Maria Luisa, PhD Candidate
Conceptual and computational modelling has continued rapid development in the past decades as it is based on fundamental constitutive physical equations and able to provide accurate analysis and prediction, however, it suffers from problems associated with computational performance, which can hinder its usage. This project will integrate fundamental physics into the training process of deep learning processes, engaging computational modelling and deep neural network, and establish a new generation of physics informed deep learning methods, which can provide accurate and quick estimates of groundwater system response. The outcome of this work will highly benefit to geotechnical engineering and groundwater protection in climate changing scenarios, for instance in the forecasting of drought.
This video explain the research in detail: https://www.youtube.com/watch?v=exewrFSnKJk